Avoiding Common Pitfalls
All models are wrong, but some are useful.
The above quote is also nicely exemplified by this xkcd comic:
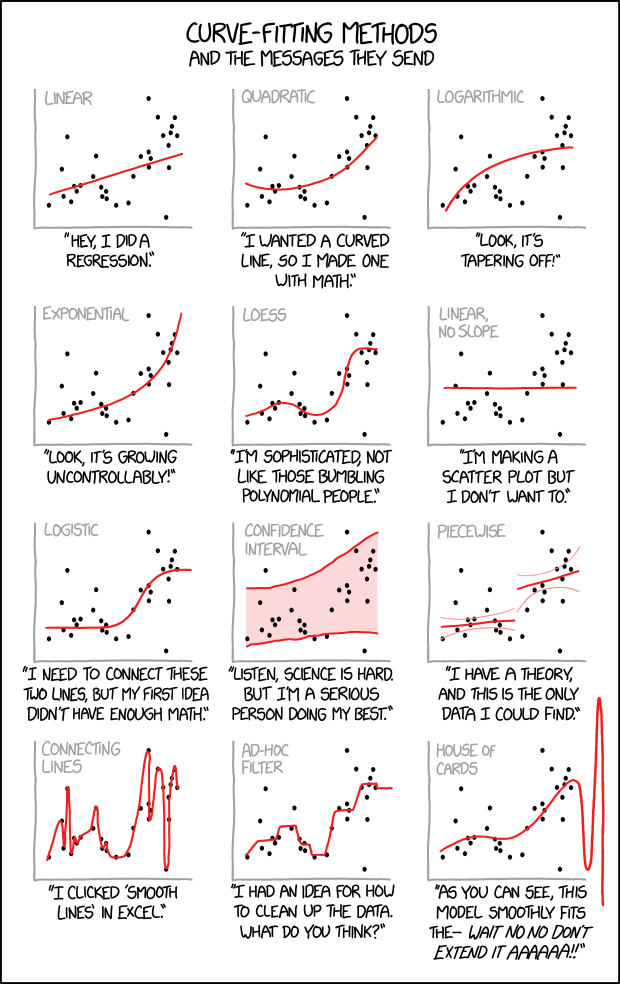
A supervised learning model tries to infer the relationship between some inputs and outputs from the given exemplary data points. What kind of relation will be found is largely determined by the chosen model type and its internal optimization algorithm, however, there is a lot we can (and should) do to make sure what the algorithm comes up with is not blatantly wrong.
- What do we want?
-
A model that …
-
… makes accurate predictions
-
… for new data points
-
… for the right reasons
-
… even when the world keeps on changing.
-
- What can go wrong?
-
-
Evaluating the model with an inappropriate evaluation metric (e.g., accuracy instead of balanced accuracy for a classification problem with an unequal class distribution), thereby not noticing the subpar performance of a model (e.g., compared to a simple baseline).
-
Using a model that can not capture the ‘input → output’ relationship (due to underfitting) and does not generate useful predictions.
-
Using a model that overfit on the training data and therefore does not generalize to new data points.
-
Using a model that abuses spurious correlations.
-
Using a model that discriminates.
-
Not monitoring and retraining the model regularly on new data.
-
Below you find a quick summary of what you can do to avoid these pitfalls and we’ll discuss most these points in more detail in the following sections.